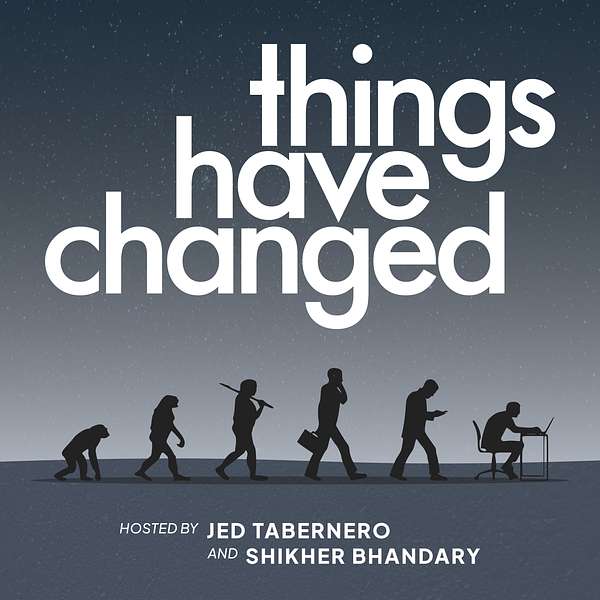
Things Have Changed
Things Have Changed
How to Build a Better AI For Your Business – with Hyun Kim
Is it possible to make artificial intelligence more accessible to companies, both large and small?
Our latest episode is with Hyun Kim, Co-Founder and CEO of Superb AI, a company that is building a platform that aims to make shipping AI models easier.
With a highly technical team, that spans 25+ research publications, 7,300 research citations and 100+ patents in computer vision and deep learning technology, Superb AI aims to utilize decades of experience to lower the hurdle for industries to adopt machine-learning technology.
With the rockstar team, SuperbAI uses deep learning AI to label and analyze images and videos up to 10 times faster than manual processes can!
It’s no wonder, their clients include not only small businesses but also Samsung, LG, Qualcomm and Pokémon Go maker Niantic.
With a recent $9.3 million financing round, Hyun and team, are looking to expand further in North America and enter Europe. And we at THC cannot wait for this future to unfold 🚀
THE HIGH-EARNING WOMEN PODCASTThis podcast empowers high-earning women to make informed financial decisions and thrive.
Listen on: Apple Podcasts Spotify
Jed Tabernero [00:00:05] There's so much data in the world, pictures, videos, text and audio machine learning brings the promise of being able to use this data to answer hard questions. The most obvious example being a machine learning algorithm recommends the best result cater to your individual profile. But it's not just Google anymore. All companies are rushing to utilize their data to build better products. It's no longer novel to use machine learning in your products. It's now an expectation. As the industry is burgeoning, companies are building their own small teams and entrepreneurs are coming into the space to solve problems of how to scale these technologies. In other words, create the small operations infrastructure. Today, things have changed. We sit down with one of those entrepreneurs that are helping create the next wave of tools for your companies. Small teams. Mr. Hyun Kim. He saw a problem with how companies were using machine learning
Hyun Kim [00:01:10] for all of these. Probably the first thing you need to do is collect data labeled data, and that takes a large chunk of your time. So I thought this shouldn't be the way it should be done. There were a lot of new research coming out from the academia that just wasn't getting applied to the industry. And I thought the bottleneck to that was the data. So they're always new research coming out in academia using standard benchmark open source datasets. But if you want to apply that to the industry, each company has to come up with their own unique data set that fits their application scenario, and that takes a long time. So I wanted to solve that problem.
Jed Tabernero [00:01:45] Ken and his team of researchers and engineers have built superb A.I., a company that is creating the new standard for Mellops.
Hyun Kim [00:01:54] Basically, we give them all the tools, the tool sets that they can use to debug their issues. So if they think their data, maybe it's too slow. We give them the tools to train our based on their data using just a few clicks. And if they think they're spending too much time on data auditing, we also give them the appropriate tools for them to automate that piece. And we give them the tutorials, give them the best practices, we give them documentations and help them use our tools to fix their problems.
Jed Tabernero [00:02:27] Whether you're a product leader or Emelle engineer, superb. I will help you execute your projects in less time. You know, the majority of my team spend more than 50 percent of their time managing training data sets. Stick around to learn how superb A.I. is reducing that time with our auto labeling and collaboration capabilities. Welcome to THC, where we unpack the ever changing technology economy
Adrian Grobelny [00:03:07] hangout with Jed, Shikher and Adrian as we tackle the industries of tomorrow.
Shikher Bhandary [00:03:13] This is things have changed.
Hyun Kim [00:03:21] It's actually an interesting event in my life back then in 2016, I was a student at Duke University studying robotics deboning division, and I was at that time more interested in robotics than A.I., for example. And there was a second semester of my first year that I remember it. I think March. And we were kind of like debating with my lab mates, you know. Do you think Lee Sedol will win or do you think Alpha will win? And it was pretty much split half and half, even among the A.I. research engineers and the students there. And, you know, like you all know, AlphaGo won. And I think I think or even among the academics, there were some skeptics as to what I can do and even more so in the industry. So after that event, so with the background, I'm from Korea, so I was born and raised in Korea, lived in Singapore, in the US. And and, you know, the game of go is really popular in Korea. You know, kids are taught how to play the game of go during elementary school, middle school. And I think everyone, every single person in Korea probably thought, you know, we said will win. And I think I think this event brought a lot of shock to basically everyone in Korea and that it basically sparked a huge movement in Korea where all of these tech companies like Samsung, LG, those guys, they realized the power of A.I. They realized that, you know, what they thought about A has to change. So they started to invest a lot into it immediately. So so after that event, I basically got. Basically got scouted from this company in Korea called SK Telecom, it's like Verizon of the US. So they started a corporate research club just for, I think, like two months after AlphaGo. And it was the same for a company like LG, like companies like Samsung and everyone. So it basically changed my career trajectory. So I, I planned to finish my PhD and maybe move over to the Silicon Valley and work for companies like Google, maybe as a research engineer, maybe your postdoc professor. But then, you know, they then basically shifted my career to become more industry focused person and eventually leading to me starting a company.
Shikher Bhandary [00:06:19] I didn't realize how significant it was that moment specifically, but we were like watching some of the videos of the tournament. And you can see, like, the inevitability in the person who lost his face is like, what just happened? This did not happen. Yeah.
Hyun Kim [00:06:40] So I don't think I don't think he ever imagined a computer beating him. Right.
Shikher Bhandary [00:06:45] Yeah. You can just see it on his face. It's like, did that just happen? And and this this feeling of what is my place in the world now, it's kind of kind of weird. Like the reason why I was able to spot that is because there are literally like YouTube comments talking about go to minute to this and you can see basically what he thought the future of humanity is.
Jed Tabernero [00:07:08] It takes a lot of like creativity. I think that was the big thing there. Right, is that Lee Sedol was, you know, as the the vice videos came out about that specific event, I watched the vice video fricken brilliant, by the way, and it educated me to the number of of possibilities, possible moves that you have it go right? Ten to ten to the one hundred seventy. Bro, that's that's I can't even imagine how many possibilities you could go from from each move, you know, and the amount of creativity for me was like, OK, there's no way that shit is going to happen with AI. But I guess I was significant moment dude.
Hyun Kim [00:07:45] Yeah. Yeah. And and just to know, fun, fun fact so Lee Sedol retired just a couple of years after that event and I think I'm not a go expert, but what I hear is there are certain like rules to go or like well known moves like opening's of chess that everyone used. But then after AlphaGo came out, you know, everything changed. So there are there there are rules that everyone, all the human Go players thought was the best patterned moves basically changed. So AlphaGo changed the basically create a breakthrough in the way humans play go. So I think AlphaGo changed what everyone thought was the best move to play at certain points.
Adrian Grobelny [00:08:42] Wow, this is three thousand years after. Yeah, I've had time to master the game, you know, all the opening strategies.
Hyun Kim [00:08:49] Yeah, yeah.
Adrian Grobelny [00:08:50] Exactly how many moves. This is really relevant because I just watched the Queen's Gambit on Netflix. Great show. And so that got me into like a black hole of just like chess.
Shikher Bhandary [00:09:01] Did you order a chess board? Because apparently that's what people right off the chess bible.
Adrian Grobelny [00:09:05] Yeah, the the downloads for the apps were just like skyrocketing for after that came out. It was really big. So, you know, AlphaGo happens you were working in robotics and then you started to kind of get more focused on it. I, I wanted to kind of figure out what was what was like the was the AlphaGo really that moment where you were like, wow, like I has this possibility and there's so much that can be done with it.
Hyun Kim [00:09:33] So it was more of like these companies started investing very, very heavily on my research and research engineering and it basically opened up a lot of opportunities for me. So before Alpha Go, I think. I think I had basically two choices. One is to become a researcher and stay in the academia or become a an engineer or industry focused researcher and work for one of those Silicon Valley companies. But after I go, a lot more companies started investing. I started launching new research labs. And I think that just opened up opportunities for me. And I always wanted to. Sometime you go back to Korea and work for work there at least a couple of years, so I decided to actually took I took a leave for two years from my Ph.D., went back to Korea, worked there for two years, and then the plan was to come back and finish my PhD. But then, you know, that didn't happen. You know, I started the company
Shikher Bhandary [00:10:39] now and better pastures for sure. So was it just at SK where you decided where you were able to maybe you were working on a similar problem where you're trying to. I guess I'm foreboding. Yeah, but you saw the issue about the whole labeling aspect of of data and then decided there could be a business application for something like this.
Hyun Kim [00:11:03] Yeah, so so I think both during my time doing my Ph.D. and also at that time to escape, I found myself spending a lot of time handling data. So, you know, for example, during my research, my my PhD focus was basically having robots learn how to manipulate objects. And that was basically based on a lot of trial and error. So like a robot would try to pick up an object, it fails and then tries to learn why it failed. So the next time it does better. So there was a lot of data gathering, both using simulations and using real robots. And also during a time that we worked on and things like self-driving, smart speakers, gaming, I, I worked a little bit on Starcraft AI and for all of these projects, you know, the first thing you need to do is collect data labeled data, and that takes a large chunk of your time. So I thought this shouldn't be the way it should be done. You know, there were a lot of new research coming out from academia that just wasn't getting applied to the industry. And I thought the bottleneck to that was the data. So there are always new research coming out and academia using a standard benchmark open source data sets. But if you want to apply that to the industry, each company has to come up with their own unique data set that fits their application scenario, and that takes a lot of time. So I wanted to solve that problem. And initially, I did some research, so research published the papers on ways that, you know, new new techniques that can enable researchers to spend less time labeling data and still end up being appropriate for train algorithms that perform as well. And then eventually, I thought that the technology is getting better and should be ready to be applied as a product. So that's when I started the company.
Adrian Grobelny [00:13:13] And. And did you start this company kind of just on the side as a side project, or did you feel like, OK, I need to put together a team, I need to, you know, put on my time, invest all my energy into this? How did you go about, you know, approaching and starting and also forming the team? I mean, you had to have you had a really solid academic background. So did you really kind of poach or like recruit academics to really, like, take on this challenge with you?
Hyun Kim [00:13:44] I basically approached a couple of my colleagues and I said, yeah, well, yeah. So we had a great team, basically, you know, after Alpha Go, companies started investing a lot into research. And basically my team had a lot of great researchers, engineers from many universities or other different engineering backgrounds. So there were a lot of potential co-founders available on my team. And yeah, I was able to poach some of them.
Shikher Bhandary [00:14:17] You were just like, you know how I work. I know how we're going to create a superb AI Team
Adrian Grobelny [00:14:28] Clever, they're clever.
Jed Tabernero [00:14:30] How was how was that education process for the name? Because when I saw it, I was like, this is this is a lot of hype, you know, like. Yeah. What's his name?
Hyun Kim [00:14:39] Yeah. So I think back then. For the very first naming idea was actually superv, superv, as in like, you know, supervised learning what that is. Yeah, yeah. So a lot of labeling. I mean, I love the A.I. that goes into, you know, real world applications. It's mostly supervised learning and supervised learning is the type of machine learning technique that requires a lot of data. And since you wanted to make that more efficient, we took the word supervised and then just used the first that super. And then we thought, hey, that that sounds a bit weird. We should change that disappeared. Right. So that's that's that's the story. It's pretty awesome.
Jed Tabernero [00:15:31] I love that story as we're kind of getting into how, you know, you build SuperbAI. I want to see, like, the first use cases, like what was the first problem you wanted to solve? And, you know, how did that materialize into the company? Because there had to have been one problem where you were just like and so much what you were talking about earlier. What was that first use case is definitely.
Hyun Kim [00:15:55] Definitely. When you collect a bunch of data and label them and then you train a model when you're on network, the accurate the accuracy of the model improves. You know, not I wouldn't say linearly, but if you have more label data, the accuracy improves. And I think it was very obvious and I think a lot of people knew that you could somehow utilize. Eh, that's not perfectly accurate, but still somehow leverage that and they bring more data, right? Let's say you have an area that's maybe like 50 percent accurate. It's not usable. It can't be deployed, aren't self-driving cars, but there's some value to it in a way that it can be used for. So maybe if you use the A.I. to label more data, maybe 50 percent of the labels are accurate. So you can only work on the remaining 50 percent manually. So that was the initial idea. So it would be basically like a back and forth thing between a and human. So human would manually label a small bunch of data, use that to train a model, and then now you have like 50 percent accurate. I use that to label more data and then a human would just go in and verify it, edit the output of the air, and then now you have twice as much data and use that to train version two of your model and then the cycle repeats. Right. So it's a very sensible idea and we want it to basically show that it works. It's cheaper, it's faster, it's more accurate. So that was our initial MBP product,
Shikher Bhandary [00:17:41] the classic example of how a bunch of pictures of trees, different trees, a tree in winter without leaves, a tree in some pine tree, whatever. Right. And then the human categorize these all as trees. And now you get the eye to kind of learn that these are the trees. So then you provide. So now these photos are labeled very specifically. Right. So now they're actually when you when you add small deviations to it, like see you you provide trees with in fall and summer and then you sure like a winter tree with fewer leaves, it can predict that. And then you're just solidifying the platform by by giving it cleaner data and more or less.
Hyun Kim [00:18:38] Yes. Yeah. I think I think that that's more or less correct. So I think a better example would be like a classical example is detecting cats and dogs. Yeah. So let's say let's say I initially label one hundred images. Fifty cats. Fifty dogs. Right. And have I learned that and and now I have a next batch of say five 500 images. Now the the A.I. that I trained with the initial hundred image batch, maybe 50 percent. Correct. Maybe 60 percent. Right. I use that to categorize the next batch of images. The five hundred images now. That, you know, the the output of the is not perfect, so a human labor or human data, a person has to go in and visualize, verify, edit the output of the A.I. So maybe 60 percent of cat images are tagged as cats. The remaining 40 percent may be misclassified as dogs. So a person would go in and change the tag from dog to cat. Now that is that takes less time than humans going in and they bring 500 images from scratch,
Jed Tabernero [00:19:57] super iterative process and a lot of man hours. It sounds like labeling sounds like, you know, maybe next to data collection has a lot of input for just really manual work. Right. Who typically does that labeling piece? Like is that that ML researcher who's spent his life studying ML? and is down there like literally labeling shit for hours or do they outsource that sometimes? And what's more prevalent in the industry right now?
Hyun Kim [00:20:25] It depends on the application. So if it's an application where little, little or no background, all this is required like self-driving, pretty much everyone can recognize cars and traffic lights. It can be outsourced to crosswalk workers. There are companies that recruit, train and manage these crosswalks, labors for data labeling. These are mostly companies that utilize the workforce in developing world countries could be South East Asia, South Asia, Eastern Europe, Africa and Latin America. And for cases where the data is sensitive or there is a lot of data privacy into the data, then the companies have to enable the data in-house so they will recruit, hire a team of data labelers and data labeling team managers, U.S. engineers and so on. And they could do everything in it now. So this is, for example, the case for Tesla? I think they I think it's known that they operate their own in-house labeling team to label their data. And also also the third case is where specific domain knowledge is required. So for things like medical images, you don't want to be like labeling medical images on crosswords. Workers like you want to have doctors or at least at least med students to label these images. So there are different types of labeling.
Shikher Bhandary [00:22:08] It's so interesting because like every captcha that you do on Google. Right. To to. Show that you're actually a human and not a robot is all traffic lights in zebra crossings. It is identify a boat like a car needs to know what a borders. But I guess it does identify the water. You know, there's a lot of data collection that they're just they've just dumped on the public to figure out. And I don't know. I've been doing that. How many years has it been that we've been using capture and just doing traffic light stuff like 15? So the data that they have is so concrete as well. It's like, yeah, it's it's kind of crazy to think to the extent to which these companies are using getting value out of just mundane activities like this.
Hyun Kim [00:23:05] Yeah, yeah. I think I think I think it's pretty unfair that Google gets the idea that it's basically free labeling, right? Yeah. Yeah. It's like global worldwide crowdsourced labeling. Yeah.
Shikher Bhandary [00:23:20] It's ridiculous because what I do is I have like this VPN set up, so it's always routing it to a different server in a different country. So every site I go in, I need to do the capture. And I'm like, how many years have I been doing this? These guys might have such a solid database.
Hyun Kim [00:23:39] Yeah. So all that data, you know, might go into Google Maps, maybe Waymo. I don't know what it was, but I think it's a huge database.
Shikher Bhandary [00:23:47] They've literally crowdsourced the data that goes behind Google Maps, which is crazy to think about. Yeah.
Hyun Kim [00:23:52] Yep, yep.
Shikher Bhandary [00:23:54] So it seems like the same. There would be a lot of variation to kind of deal with. Right. A lot of noise that you might be dealing with. Now you are a company that works. It works with clients and other companies trying to better curate their data and get insights out of it. Right. So how does that process look like? Because companies have different data, right. Or do you stick to one line of market segments like, say, you just look at, say, for example, discard. So now that the algorithm that you have knows what cars are and are is a bit more in tune with the segment that you're working with,
Hyun Kim [00:24:45] that's a good question. So a lot of the aid that we use on a product is designed to either automated enabling or automate data, keep seeing or data cleansing or any of these, you know, processes that I just talked about. And initially these models or these AI's that we have in-house that's trained on a large database of open source data sets that and oftentimes these cover like common objects. For example, one of the most popular opensource that's called CoCo Dataset, and it's an acronym for Common Objects in context. So, you know, these are like hundred different objects, like cars person, you know, like baseball cup, bottle, whatever. Right. So initially, initially, the models are trained on these officers data sets and the. And in addition to that is the ability to easily fine tune and customize our aid using a small piece of a small portion, a small portion of our clients data. So you can think of it as like we have a route or a base set of our models. And based based on each client's data, it will basically kind of evolve and become more customized to each client data. And and and our core tech is being able to do that without any human intervention. So it would be pretty easy to do it with human intervention, but it wouldn't be very scalable as a business. So we need to be able to do that on fully autopilot. And it's something called Auto ML. So that's a part of machine learning that makes the A.I. learn by itself without any human intervention.
Hyun Kim [00:26:49] Like, where do you start to learn their database, learn the way their operations are going and find inefficiencies? Or you can 10x your processes by, you know, using our product here, like where do you start when you just get a data dump of all this information from the company and what they're working on?
Hyun Kim [00:27:10] Basically, we give them all the tools for the tool sets that they can use to debug their issues. So if they think they're data labeling is too slow, we give them the tools to train our base on their data using just a few clicks. And if they think they're spending too much time on data auditing, we also give them the appropriate tools for them to automate that piece so we don't actually go in and do the work for themselves. We give them the tools and we give them the tutorials, give them the best practices. We give them documentations and help them use our tools to fix their problems. Now.
Shikher Bhandary [00:27:54] Interesting and, you know, I come back to that that thought that, OK, you create like a skeleton, like a framework, and then you kind of deploy it to, you know, where it's where you where you see fit, whatever fits the metaphor, I guess that client or so. So is this the gap, you know, within the market where everyone wants a scalable. Technology. They are sitting on mountains of data, but they need a way such that they can scale to their specific needs and as a business for you, you don't want to be reliant only on one client. You have many clients. So you kind of need to build a portfolio of, I guess, models that works better in different scenarios.
Hyun Kim [00:28:51] I see largely two groups of clients. One is very high tech companies that already have so much data. They have the engineering resources and they just want to be able to increase the accuracy of their models to the extremes. Right. Maybe they are a driving company competing with Tesla. I don't know. It could be I got, you know, physical security, CCTV camera that's competing against others based on their prevision accuracy. And the other group is. Actually, oftentimes traditional industry companies that have the data but don't have the resources to build models, and I think interesting to my surprise, both groups of clients saw value in our custom customizable ehi that's built into our product. So I initially I thought, you know, being able to quickly train a custom model and use that to accelerate the data labeling process would be more beneficial to the second group of people that actually don't have the resources or the knowledge to train themselves. That was the case. But it was also interesting that these high tech companies also found value in that, because it's like they know how to do it if they had the time, but they don't want to spend time on it. So they want to spend 100 percent of their time building better algorithms, building better, you know, whatever better self driving it. Instead of spending time on ways to accelerate or automate their data labeling, they have a pipeline. So I think I think I think these companies will will go into that in maybe a few years. But I think right now their focus is 100 percent on improving their model accuracy. For example, instead of spending less time, less money on hold, data labeling, data management pipeline. So.
Shikher Bhandary [00:31:08] Of something you said that just triggered that the thought in my mind was like a good friend of mine who is really equipped with the skills he works as a A.I. architect for Google, but he does not he's not in Google like he's he's part of this company that Google. Outsources a certain amount of. Work beat, you know, a certain amount of data that he comes in and he's working on those models as well as a contract for the company, so they have like their own little deals with companies because they want to focus. They are research teams on certain aspects of the process.
Jed Tabernero [00:32:02] What are the highest risks within, you know, the machine learning deployment pipeline right now because of issue tracking, because of the ways that you're able to interact with different parts of the normal process, you're able to determine a more accurate timeline for when this is going to get deployed. The problem, essentially, that you're solving is how fast can we get this to deployment and how easy can it be?
Hyun Kim [00:32:27] Like, if you think about software engineering, there has been that DevOps movement for so long, right? There are so many software engineering tools things, and it's not just toys, but more like the way way of working, like they're like core principles to evolve. Right. And I think with that came, you know, companies were able to better estimate how long it's going to take to build something in prototype that and also be able to better collaborate with cross-functional teams, you know, make their products more observable, make their products more measurable, you know, automate a lot of processes, you know, continuously improve multiple iterations of their engineering cycles. And I think that something similar to that should happen and is already happening and machine learning so, so similar to whatever it's called, MLops. And sometimes the data part of the stack is called data ops. So I think I think that change and shift in mindset should happen. That's the first thing. And I think the other thing is there's no no canonical Thek and machine learning. So for software engineering like you have, let's say you want to use source code, source code management, you are either going to use GitHub, Gitlab, or BitBucket right, or if you want to use cloud, its either AWS, GCP or Azure. So there's that stack that everyone uses. But in machine learning and data labeling or data management, there's no that's stack yet. I think the machine learning, machine learning, MLops and data market is a bit too early. There are so many companies coming out that, you know, these these people, they don't actually it's very hard for engineers and researchers know what kind of tools are available out there, which tools are going to solve their problem, or which tool integrates or goes well with which other tool. And I think, you know, companies or these engineers will start to pick up or learn, you know, or start to build these canonical stack. And then I think then we'll have more visibility, more measurability. And then I think the whole machine learning projects getting shut down, midway heading, that will decrease as time goes on.
Jed Tabernero [00:35:13] And I'm glad I'm glad I read that that link that you had put in for towards data science. Yes. I didn't understand 90 percent of it, but that's fine. I understood the main point, which was that you're building the infrastructure to be able to provide these services and to scale. That's really the thing, right? It's similar to what you're doing with your company as well, is that you're trying to scale, you know, MLops like we need to have teams that are able to do certain parts of the processes in order for this to be well adopted. You know, across the
Adrian Grobelny [00:35:42] board, as we're just discussing and you're bringing up you know, there's so many companies going into this just on this podcast alone, which has only been around for a year. We've had so many I guess we've had I in journalism, you know, finding ways to create frameworks for and writing. We've had a I for autonomous driving, which is kind of the mainstream. We see it everywhere. All these companies are working on it. We have even had a first synthetic media just creating videos, a person and making their Lip-Sync. Yeah. So, you know, we're just seeing all of these applications come in and, you know, you're you're a founder. You're the CEO of your company. You're you're basically the leader of your whole team is looking to you as guidance, as a leader of what you're what the direction of your company is. So as you kind of look forward of where you see this market going, like what is the the TAM like, what is the market that you're basically working to?
Hyun Kim [00:36:47] I don't have a number in mind but I'm thinking about the you know, the market landscape in general. There are a lot of different sections or portions of the machine learning development cycle industry that, you know, many different companies are tackling. So, for example, there are companies that focus on data collection, some companies that focus on their labeling on training, data analytics, model analytics, model deployment, infrastructure metering, and all of these know so and so forth. Right. And right now, the. Mellops market is very early in their stage, and so these companies are tackling very niche problems, right. For example, we started out with, like I mentioned, the very, very early earlier part of this podcast that we wanted to automate the beta labeling piece. Right. And as these companies, including ourselves, tackle the one initial problem that started out with, it's going to expand out naturally. And I think in a few years there will be companies that will start to overlap. Right. So, for example, maybe a data collection company could start to overlap with the labeling companies or data model training companies would start, you know, overlapping with model deployment. Right. As they as the companies grow, as they find more clients or markets that they want to tackle. So these overlap will happen. And I think at that point, a lot of market consolidation will happen. Right. Companies acquiring each other, becoming these massive companies going IPO and stuff like that. And given that future in maybe five years down the line, very high level, I try to think very strategically like which companies should we be going together with? Like like, for example, the whole point of ML Ops Data ops is being able to give a lot of tooling toolset for these engineers to stitch together and automate all of their pipelines. So inherently, there's a lot of integration components, too, and a lot of data. So trying to think which players in the market should we be integrating with? Should we do partnerships with? I think that's a high level strategy question that's on my mind always. I don't have the answer to I mean, if I had the answer, it would be a bit in there already. But yeah, it's something on a very high level. It's a very important question for me, you know.
Shikher Bhandary [00:39:39] Yeah. It's such a hard thing to kind of digest to see what how niche a product can be and how big the size could be. You know, like we were just chatting about how DocuSign is a e-signature company. Right. And it's 40 billion dollars, 40 for zero with
Jed Tabernero [00:40:02] Just to sign shit.
Shikher Bhandary [00:40:03] Just so like when people when it just came on the scene, people are like, this is just an e-signature company. There's nothing different. But then the whole point was we are going to digitize the agreement. And companies across the world, people across the world. Have agreements. We are going to digitize that, so it's like small niches like you just mentioned, like, you know, there are many stages to this, many levels to this to the MLops procedure process that companies go through. Right. That you work with companies on each could be its own category defining segment. You know, like it's just crazy to think how, like the whole world is. So, like the. The reach, you can't really quantify certain things because you could go Wadle and just, you know, have that whole category. So it's interesting to think about.
Jed Tabernero [00:41:10] So I mean, as as we're talking about, like different niche parts of the industry and integration between products. Right. Supervisor SAS platform. Yep. So there's a lot of opportunity for you and your team to be integrating with products as you're kind of doing that already. Right. Toward certain parts of the procedures. So I guess like what are your your strongest suits, strongest parts of the the sweet product, like what do you show off to everybody? And, you know, when I look on your website, looks like it's labeling, but tell me if I'm wrong.
Hyun Kim [00:41:51] There are a couple of things, though, currently that the two things that I like to emphasize or one thing that I like to emphasize the most is the labeling automation, and there are a few pieces to it. So no one is the labeling itself. So, you know, initially our I was only able to recognize maybe a hundred different object classes. Right. And now it's evolved using automobile or a couple of other advanced techniques, and now it's able to adapt to each client's data set. Right. And that only takes maybe a few hundred images, a few hundred labeled images and maybe I think it's actually four clicks. And one hour after you will have a trained model that's fine tuned to each client's data and the clients can immediately use that to label people more of their data. So that's that's what what we call custom auto label. So the initial auto label was that the model that was able to detect different underclasses. Now the customer label is being the customized to each client data. So that's that's one piece. And the second piece is when are custom labels the clients images? It not only outputs the labels, but also outputs its measure of how difficult the image was to label and how difficult each object within the image was. Difficult to detect. Right. So that's very useful for data editing. So instead of having everyone, like all the crosswords workers go in and manually visualize, verify, edit all of the output of the air we can proprietor's and sample based on how the thought the image was difficult. Right. So let's say you have, you know, a million images that you want to label instead of manually inspecting every single image or instead of sampling like 10 percent or one percent of the whole dataset, you'll be able to more intelligently select which ones to audit. Right. And these are usually very complex, dense images where there are a lot of different objects in the scene. Could be a lot of conclusions, could be blurry or dark images. It's not it's not actually 100 percent interpretable or like we don't know why the A.I. thinks this image was difficult, but for for some reason it was difficult. And if a human user goes in and clicks on these images, it usually is very, very dense. Difficult. Yeah. So that's that's that's what it's what's called uncertainty, estimation technique that we baked into our auto labeling product. So combined, combining one and two, we can have our users very, very quickly labeled data and all that data and use that to train their models.
Adrian Grobelny [00:45:00] So, yeah, I wanted to dove really quickly into the clients that you're working with, the companies, you know, you have the high tech ones and the ones that are just getting into using I and their businesses. And also, you know, you're working with companies all across Asia and the US. What's how has it been like working with these different companies? How do you figure out what's the best approach to work with? Do you have a sales team? Are you just reaching out to these companies yourself and saying you're head of growth and also have the CEO hat on what's
Hyun Kim [00:45:37] so early start? So we have two offices, one in California, one in Seoul, Korea. So initially, you know, like you mentioned, I put on the head of growth hat and reached out to the US customers. Now we have a very solid team in the US soccer team. As for go to market sales, marketing of that Korea, we do we do most of our product engineering in Korea. There's a lot of. Engineering talent in Korea that we can utilize, so we do that, and in Korea we also have a go to market team that's more focused on the iPad, starting with Korea and then expanding out to maybe in Japan and Singapore, maybe South Asia and so on. Yeah, yeah, yeah. So so the cross border cross Pacific operation is an interesting challenge and it's one that I'm enjoying. It's interesting. Problem solved. So like, how do you have your product team think with your U.S. team as often as possible and how like the product team will deliver the product roadmaps, the the go to market team, the US will deliver customer feedback to the product team. And I think it's an interesting challenge. But with with the pandemic, everyone going remote, it's not very different from all these companies in the US. No.
Shikher Bhandary [00:47:05] So, like. We are fascinated because, you know, the three of us are from just three different parts in the world, right. So how is how are the interactions between these executives, business leaders that you interface with in the US versus Asia? And what's the direction each is taking with regards to this this technology? As I was reading some articles where it's like double the amount of tech that is within our daily life in the Japan, South Korea is just so much more than what an American sees. It's it's staggering, you know, so the the go to market is so much more quico. They're. So, yeah, I would love your thoughts on that difference and I guess just looking forward.
Hyun Kim [00:48:00] Yeah, so that's very interesting and it's a learning experience for me as well. So for example, I see the US market is definitely more mature in terms of mahlab state ops. I think, you know, Silicon Valley companies are driving the end of the world. So, you know, inherently they're more mature. I think they're maybe, you know, three or five years ahead of what's being developed in Korea or in APEC. So so. So when I approach our clients or prospective clients and us and Korea, their response is very different. So, for example, if I go to our U.S. prospects and do our demo, tell them our value pops, they immediately get it. And are questions like how much is it or how is it different from your competitors?
Shikher Bhandary [00:48:55] Classic. How much do you want?
Hyun Kim [00:48:59] Yeah, and compared to that, like in Korea or in APAC, it's more like. They don't they haven't thought about this kind of product yet, and sometimes they don't even know that they need something like this to better or make their machines more efficient. So it's more like we're educating them rather than direct sales. So that's that's one thing that's different. Also, you mentioned something about the the technology adoption in Korea is much, much faster, I think. I think it's true. But so the US is just this tech adoption in, say, Silicon Valley as far as faster than Korea. But on average across the US, I think Korea is faster. So there's that difference. And Korea market is relatively small compared to the U.S.. So I think I think within Asia, within APAC, we're always looking to go outside of Korea. So immediately, right next to us is not Japan and Singapore, like you mentioned. And then I think there is a lot of untapped opportunity and for example, South Asia or even the Middle Eastern region. So potentially that's that's maybe our third office location.
Jed Tabernero [00:50:25] And if you wanted to get your thoughts on the future of this space. Right. The space that's that's creating the MLops engine, you know, any predictions, any, like tipping points that we're seeing beyond, you know, Lee Sedol loss cry. But, you know, what is that next tipping point and where do you see it going forward?
Hyun Kim [00:50:46] Yep. So I haven't read a lot of research papers recently, obviously. But even even given that I do see this thing called self supervised learning, it's almost like a buzzword or some might say it's a hype, but I think there's something to it. So for for the listeners that might not be familiar, so supervised learning, like I mentioned earlier, is based on a pair of data. So you have the raw data, image, video, text, whatever, and you pair that up with what's called the label. Right. So for images, it could be a tag saying the cat or it's a dog. Right. And you use that pair to train a model. Now, substories learning is its its. The accuracy is much, much higher than other methods, like unsupervised learning, where you learn without the label piece, but obviously you need to create the label. So it's very expensive. Now, Self-supervised learning is an interesting concept where you actually create the labels using the raw data itself. So you're basically generating the labels for free. So for example, for example, let's say you have an image of a cat, maybe you rotate it 90 degrees. And give it to a machine and have it learn how to predict or have it learn to output, how to correctly position or rotate the image back to its original position, or or maybe you you cut up the image into three by three or four by four grid and mix it up. It's like like a puzzle and have the eye try to reorganize the tiles so that the the you get the original image so you can kind of see how the label is free because you, you manipulate the original image and that's the label and using that are self supervised learning framework. I think. So the AI model is actually learning a more abstract concept of the world. So instead of being able to detect cars with an image, it's being able to learn or abstractly conceptualize what a car is. So, yeah, so it's it's making some breakthroughs, I think from mostly from research that comes out of Google, Facebook, all these guys and I think. US as a startup, it's hard to create very original selves for supervised learning, breakthrough research ourselves. So I think it's our job to translate a lot of the research that comes out and put it into our product so our clients can use it to better accelerate their machine learning development management pipelines.
Jed Tabernero [00:53:58] That was a beautiful ending. You know, wanted to give you the spotlight and see what you will to our guests and where can they reach out to you work and learn about superbAI.
Hyun Kim [00:54:09] Yeah. So we're supposed to say we provide a data management platform called Superbia Suite. If you are having trouble labeling data, if you think you're spending too much time managing data, feel free to contact us. You can visit our website at double double, double dot. Superb Dash eight dot com. You can search on Google. Hopefully it's the first one that appears on the search results. Yeah, and there's a contact form to fill out the form and will reach out to you.
Jed Tabernero [00:54:41] And that's how we met. OK.
Hyun Kim [00:54:43] All right.
Shikher Bhandary [00:54:48] Hey, thanks so much for listening to our show this week. You could subscribe to us. And if you're feeling generous, well, you could even leave us a review. Trust me, it goes a long, long way. You could also follow THC, @THC_POD on Twitter and Linkedin. This is Things Have Changed.