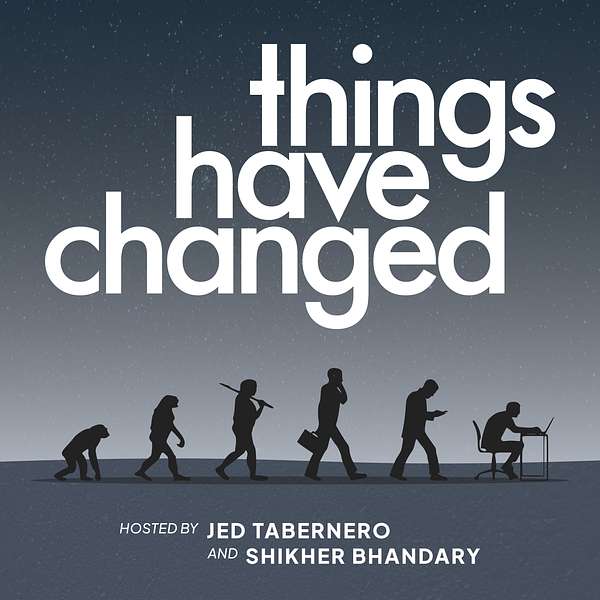
Things Have Changed
Things Have Changed
How AI is Redefining Legal Workflows with Bryan Lee, Co-Founder of Ruli AI
Today, more than ever, industries are turning to tech to address inefficiencies that plague day-to-day operations. The legal industry, often viewed as one of the most traditional and slow-moving professions, is undergoing a silent revolution. Lawyers, known for their meticulous review processes and reliance on institutional knowledge, are increasingly overburdened by repetitive tasks like document review and answering the same questions multiple times.
Ruli AI, co-founded by Bryan Lee, is at the forefront of this shift, leveraging artificial intelligence to redefine how lawyers work. On Things Have Changed, we recently hosted Bryan Lee, co-founder and CEO of Ruli AI, to discuss how AI could tremendously boost efficiencies within the legal space while giving lawyers the tools they need to focus on strategic, high-value work.
Ruli AI: The AI Partner for Lawyers
Bryan’s career journey is far from the typical one - going from big law to launching an AI startup. After spending years juggling the demands of capital markets law, in-house legal counsel, and AI development at Google and Meta, Bryan observed a universal pain point: lawyers spending countless hours on tasks that could be automated.
“Imagine if lawyers had an AI partner that could handle repetitive tasks, like summarizing contracts, reviewing for inconsistencies, or even answering basic questions,” Bryan explained. “That’s where Ruli AI steps in.”
Ruli AI offers a legal platform with two primary components:
- Legal Hub: Automates intake processes, answers FAQs, and centralizes organizational knowledge for seamless collaboration.
- Copilot: A personal assistant for lawyers, capable of summarizing documents, conducting research, and providing clear, actionable insights.
This dual approach makes Ruli AI a trusted partner that helps lawyers focus on what they do best: solving complex legal challenges.
The Legal Profession’s Efficiency Problem
At the heart of Ruli AI’s mission is the drive to solve a problem that plagues the entire legal industry: inefficiency! The numbers are staggering. In a typical in-house legal team, a single lawyer might support 100 or more employees, resulting in a backlog of unanswered questions, delayed document reviews, and unproductive hours spent on repetitive tasks.
Lawyers and AI are an Ideal Fit
Ruli AI shows that AI & Law is a perfect fit. There is growing consensus that at least in the Legal field, AI is being viewed more as an Aid, and not a Replacement. By automating routine tasks, AI can empower lawyers to spend more time on complex cases, strategic thinking, and client relations. This technological shift allows legal teams to work more efficiently and cost-effectively while maintaining high-quality services.
THE HIGH-EARNING WOMEN PODCASTThis podcast empowers high-earning women to make informed financial decisions and thrive.
Listen on: Apple Podcasts Spotify
Imagine this a corporate lawyer drowning in document review, spending weekends, combing through contracts, looking for inconsistencies and responding to repetitive. FAQ's. It's a grind that feels endless. And it's all too common in the legal profession.
Bryan Lee:At Google I think early on it was literally like two lawyers when the whole thing started out. I would say the problem starts a hundred to one, like that's where the ratio where it starts to break down for an attorney. if you don't have a good filter coming in, that's where that problem really exasperates
that's the spark behind today's conversation with Brian Lee. Co-founder of Ruly AI. Brian's journey from lawyer to tech innovator at Google. Has uniquely positioned him to tackle one of the legal industry's biggest challenges. Inefficiency.
Bryan Lee:were trying to reimagine legal work with an AI teammate. That's a North Star.
In this episode, Bryan shares how his AI powered solutions are helping lawyers reclaim their time. Whether it's streamlining document reviews. Or just automating FAQ's.
Bryan Lee:So we have a legal platform that creates an AI teammate experience where lawyers can pair their knowledge with AI and helps you automate some routine. Tasks like answering legal questions, as well as you have this copilot partner that helps you with, summarizing documents, interrogating, asking questions of documents.
Stay tuned to learn how really AI is redefining legal workflows and hear Brian's take on the future of AI in law.
Shikher Bhandary:Talking about just lawyers, right? And the legal space. So a friend, a really close friend of mine who works within a tech company. So in house legal counsel, he was telling me how on an average he's spending about 15 to 20 hours. On the weekends, reviewing cases because the legal team is small. He ends up spending hours, like 80 hour weeks, almost every week working through contracts not creating it, just like combing through these documents and looking for errors and inconsistencies, basically document review. I know the three main, Things, three main buckets of a lawyer's job is drafting, review and research.
Bryan Lee:You nailed it.
Shikher Bhandary:Yeah. I was just talking to him about it yesterday and he was just talking through some problems where he's I'm doing so much review that we made a pitch and we now have external partners, external companies, to take some of the excess workloads. From him, but even then he's working these long hours, right? So there are potentially just a ton of efficiencies here because when he was talking about document review He's if we had a database and if it is fine tuned really I think this could be a great place for driving those technological efficiencies that we are here today to talk about right? So now imagine if he my friend had a way You to leverage an AI law partner to take on some of that repetitive work, right? Document inconsistencies, summarizing key points some fixes here and there, flagging issues. It would really make his job easier and more efficient. So that's where our guest today, Brian Lee steps in. Brian is the co founder of Ruli, a legal AI company. Brian, great to have you on.
Bryan Lee:Yeah. So I really were trying to reimagine legal work with an AI teammate. That's a North Star. I'm classically trained as a peer engineer first, and then a lawyer went to law school, did big law. Doing like capital markets, securities law, basically. And then did a little bit in house doing a lot of reviews, contracts and whatnot at General Electric. I wanted to get back to my first passions in tech and then just lucked out a little bit and I'm joining this stealth project at Google, which turned out to be the Google assistant team. So I was able to work on AI, like early days the voice assistant when I came out, it was on the Google assistant home team versus business roles then moved over to the product side. And then'cause of my background in legal, a lot of the work shifted to product trust, safety, private privacy where you work, where you're developing these feature sets within products. But a lot of it was also working with like internal in-house counsel teams privacy teams, policy teams, et cetera, to make those features right. And then that also led me to working on early. LLMs at Google, like Transformers, which were invented there. I was actually using them on the ads sort of privacy safety, brand safety team. Yeah before, so this opening eye explosion
Shikher Bhandary:When was this, if I can ask, like, when
Bryan Lee:yeah, it was like I joined that team 2019, 2020, 2021 effectively, right? And then 2021 was when the. So sort a chat, UT explosion came out. That's actually when I actually left Google to go to meta. I then was working on augmented reality glasses. You probably saw some of the that's my co-founder, CTO. He was also on that team effectively, and he was a machine learning engineer. And that's his background and he's built things like trust safety models as well. Interesting enough that LinkedIn and other places also done a lot of machine learning work at Airbnb and AWS before. And then that's how, the team came together. You both left, you find each other afterwards through the YC matchup program. And then we started really from there earlier this year. And we, but we really focused on, cause, cause he had similar experiences working with the house from the engineering side, like in house legal. So we, we realized that like outside of a lot of contracts with there are, where there are tools, often people are reviewing sales contracts and there are tools for that Salesforce, connects to an ironclad and like a CLM is what people call it. Contractor contract life cycle management. I'd say some CLMs are really. Like ancient, they're basically just Google drive or SharePoint. They'll do anything. Some of the more modern ones are saying we can help you review terms and things like that. Usually it's still very hard coded to a template. So I think where people get tricked up is when it's what they call third party paper, or it's something that's not the standard, your standard sales contract, people are trying to renew a review or like procurement or contract, obviously. The contracts of the other party typically, and that's where people get tripped up. But then we also realized that, like all our engagement with most, it's council was like just through email pinging all this stuff because there's all this privacy review that has to happen for a P. R. D. Level engineering design docs to post ship to reviewing P. R. Statements like post launch. Marketing, all that stuff, right? And a lot of it's driven by, not all privacy is one big factor, usually. But it's just because like now there's so much risk management across this product development that there, there's no tool for that. And sometimes there's a lot of FAQs and whatnot. That's actually what we built first with Ruli. And that's what we were funded on. That was our prototype, which was like we, we built, you think of it as an AI paralegal that sits in. Slack or Microsoft teams or email and you can like paying this paralegal first paralegal really first and it can answer like the standard process questions or check on like light level things for you for a pilot. Right now, we're automating some lower level compliance work effectively. We're just reviewing the content that comes in and in certain conditions, we're auto approving it.
Shikher Bhandary:it's pretty incredible that you have sat in engineering, big law, in house counsel, and then product management, right? So you've got like this, literally like this set of skills that is probably super important for the role that you are leading right now, which is co founder, CEO of truly a legal startup. At least in house legal counsel, a lot of the work, maybe 60, 70 percent of the work is actually just responding to FAQs, like questions that are asked all the time. So that's number one. But also realizing that there are tons of additional efficiencies here in the space. that you can just have maybe an AI law partner to help one with. Is there like a specific case, specific instance that you remember in either Google or Meta where you actually thought, hang on, this is far too process heavy.
Bryan Lee:Yeah, I think that's a great question. I remember back at Google initially when we're this like assistant Google home team, and then they green lit this very large effort to build out all of consumer hardware from there. And then that's a side that I slotted into like the product area I slotted into afterwards and like the teams overnight went from, like a 500 person team. 5, 000 try temperature directory. And so it was actually me and a legal director because I was at the time running product strategic deals team. A we would just get questions all the time. Just flooded as new people came on and they were like do I need to sign a DA to talk to this person? When does legal need to review X, Y, Z. I had to write a policy guides with the legal person to say this is what slots in on the business side or products that it's what slots in on the legal side. This is when you, here's like things we've built that are routine. Check this thing first and then go into this section. We have to like manually assign. People have to go in and there's like a light macro here, but you basically have to, the legal director to go in a few times a week and just figure out what she could answer herself and then what she could basically assign to a team member with some context. Actually, that was a lot of inspiration for the first. Part of our platform
Shikher Bhandary:were the first AI partner
Bryan Lee:I was like, we're fashioned up the spreadsheet. And ironically, it's crazy. This thing is still in existence. Like Google to run a lot of this engagement in this part of consumer hardware is what I found out.
Jed Tabernero:pitching to them soon,
Bryan Lee:yeah. We've heard still some people there. They're looking at things with Gemini, which is a different topic. There is low level work where like the lawyers are, if you think about current state, either the lawyer who needs to review it is not getting to it, and then sometimes the business or engineer marketing just has to run without even any legal guidance just make timelines anyway. So you're really a current state. It's actually. I don't think, I think people get caught up on the error rate a little bit in an isolation box, but I always think of it as what's that human error rate today too, right? Today it's clear that lawyers also make mistakes. I remember, in my experience as a lawyer, I made mistakes. Also, I worked with a lot of in house counsel because I, have that legal eye, I would call it. But even if you don't, a lot of people were. Pay attention to detail. We'll see their typos, their mistakes, right? Because, volume spikes, but spikes up. And then during certain times of the year, people are rushing like
Shikher Bhandary:Yeah.
Bryan Lee:Of people too. And so I think the benchmarking question is really important on like people keep chasing for some sort of mythical a hundred percent accuracy.
Shikher Bhandary:What was the size of your team? The organization went from 500 to 5, 000. How many lawyers were actually now responsible for those 5, 000?
Bryan Lee:I can't remember the exact number and I think At Google, it's probably better staffed, but like early on it was like, it was only literally it was like, I think early on it was literally like two lawyers when the whole thing started out. It was like, and then they expanded out to other lawyers within the team. But the point was literally like you had two or three people, and then for this entire product area on the Google home side, maybe one person on like the assistant side and that's how all these things start out. And then, you get to this inflection point and it does expand out pretty quickly to more support, but I would say 200 to one or the problem starts a hundred to one, like that's where the ratio where it starts to break down for an attorney. if you don't have a good filter coming in, that's where that problem really exasperates, right?
Shikher Bhandary:that's a great. explanation of the problem space where, you have these multiple orgs, suddenly they have different requirements and two lawyers in the in house council is not going to cut the just varied requests, but still similar in many ways.
Bryan Lee:Yeah. You'll find right now, cause we're targeting more on the mid market and kind of late stage startups for early customers. You'll find that like our sweet spot is like three to five lawyers where we find the problems really start compounding and then up to 10 more team. And then at that point they have The muscle memory as well as some resources to write the knowledge documents. That they can put into our software to make that makes things repeatable. But it also takes this effort where, maybe your hair's on fire and you have to actually find a little bit more time or maybe, carve out a little more time so you can document some of that to tell the AI what to do. Sometimes the hard part is just convincing folks that like, Okay. You spend literally a few days, not even a few hours a day for maybe just a few days talking a few of these things. And it can be in plain language. I think that's the power of AI now. some lawyers, older lawyers have PTSD of integrating with last gen's tools where you have to spend six months with a vendor and Okay. Try to do machine learning, some sort of like hard code, programming rules, no code for your own stuff. And then they have that PTSD, but I try to show them that, look, you can literally just write a Q and a document the way that you would to a person on team, just give us existing policies that exist that you, you try to, share among the team already. But nobody's really reading them or misinterpreting in the wrong way. And that's the sort of like plain language document. When you onboard, really, it's think of it as you're giving it, onboarding documents the same way you would to somebody new that joined your legal team, right? And that's just more like human intuitive way of looking at it. And then I would say that we actually got a lot of interest after we launched the initial part of our platform on we call it legal hub. This legal efficacy part that we just discussed. We actually got a lot of interest for product we didn't have at the time, which is copilot. So we like you're a tragedy for lawyers because that's actually a very more one to one relationship of assisting the lawyer themselves. And so we just launched that in October. And so now we have both sides of our platform. The way you think of it is co pilots like this personal assistant for one for each lawyer. And then the legal hub helps scale your support knowledge to your clients internally at the company. And that's really how our Two halves come together, but it's all powered by a knowledge base that you can basically add files in that you're making the experience work for you.
Jed Tabernero:Brian, do you find that your target customers have a pretty robust knowledge base? Or is that something you have to force them to go and develop and create?
Bryan Lee:Yeah. It's very interesting to hear that. I think You'll find that as I said, around the three to five lawyer base, people have this repeatable knowledge. They've probably taken a step of I remember back when I was doing in house counsel work, I'd get the same questions even. this is like over 10 years ago, I would put things In a word document, and then when I get emails coming in or things like that, I just copy paste the answer. and this is actually quite common today still, for many attorneys,
Jed Tabernero:Oh yeah.
Bryan Lee:when I uncovered it, so I realized oh yeah, I keep a word document without making use of it, I just copy paste out my answer. And so you'll find that around again, that kind of three lower range people have now repeatable documentation, they want to put that in and that's, that does exist, I think even, but for copilot itself, it's such a good assistive tool that we've had people who are like solo GCs that are interested in this because it's, if you add just Your templates or your, things that you're already working with that can make it helpful, but it can work without knowledge base to we just have this, fine tune copilot with some like legal shortcuts built on top. And so you'll find usually there's no issue there, I think on the larger enterprise side, what we've discovered is. It actually manifests in a different way. You'll find some companies that we've talked to are like, oh yeah, we have all this documentation. We actually have talked to other AI vendors. We don't like. How they're implementing it because they give us standard model answers. We actually like what you have because you can put in the documentation and then it runs that way, which is actually how we run as an organization. But then you'll also find there are some legacy companies that I've talked to where But we have 200 lawyers and actually like the problem, it's we don't even know how they're answering it like themselves. So there actually is no organizational consistency. And so that's actually a different problem at that point. That's actually a, like change management. Like you have 200 human lawyers that actually don't have a SOP and everything's in their head and they're actually like, Just they're just it's based on their experience and like what they like agreed upon with the business unit that they support and there's no documentation even for themselves, which is like a greater organizational problem where that's why with those organizations, and usually legacy companies like. Allure leaves, all the knowledge is gone they don't know, and a new person comes up and they have to almost start from scratch, re engage the business engineering teams and figure out what to do, but that's something I don't think that we are trying to solve today, but maybe AI in general I think will improve. We have systems in place that retain that knowledge for a customer. I think that will make a difference for enterprise down the future. We actually privacy is a big issue. So we actually do like a unique instance of our product for each customer so that it learns. More specifically for that customer, we don't train a custom model, though we're using open areas like reasoning model with like our own sort of machine learning level rag on top for each customer and that's how we're addressing that issue as well.
Shikher Bhandary:Jumping into the Rooley solution. You have pointed out so many inefficiencies in the space from a small, medium, and large law firm or large company with legal counsel in inside of them. So can you explain us, can you peel the layers of what Ruli is now focused on what the solution is? And you had mentioned the co pilot and different verticals within Ruli, but can you give us a broad overview of what Ruli AI is currently designed to do?
Bryan Lee:Yeah, that's right. So we have a legal platform that creates like an AI teammate experience where lawyers can pair their knowledge with AI and helps you automate some routine. Tasks like the answering of legal questions, for example, at that intake stage, as well as you have this copilot partner that helps you with, you might be summarizing documents, interrogating, asking questions of documents. You can do light legal research on it as well. We also have the ability to go to external search. And we have citations built in. That's something new that we've just launched that allows you to zoom into a specific section of a document that we've cited and that really eliminates it mitigates that, that hallucination factor to give you the ability to audit really quickly how we come up with responses. And we've also built this. Interesting new feature today called magic prompt that launched yesterday. Cause a lot of lawyers have this. Like page problem, which is why they have trouble using co pilot for example, or even chapter BT. It would magic prop. You can type in almost like some chicken scratch or an idea of what you're trying to accomplish. And then we actually expand the prompt to be more comprehensive, robust to get you a more legal oriented answer that you're looking for but really we're focused on this you call it like the frontline. Support that in house council teams deliver to their stakeholders,
Jed Tabernero:It's interesting because you keep mentioning that kind of your target focus is these smaller firms. But I guess me being in a large company with quite a bit of resources, it just seems like something that would be extremely useful for us because we also have a ton of lawyers, right? But our correspondents are all via email. There is no tool that I can consult. There is no FAQ that I can go to when I have legal questions. The stuff that we work on are typically PNC stuff, right? Privilege and confidential stuff. And we have always questions to legal counsel, right? We have external legal counsel for some for tax reasons, especially we reach out a ton to external legal counsel. And it takes a while to get an opinion back, man. And dude, it's not, We have so many questions that there is like this massive log that they'll share with us. Hey, listen, we got actually 85 questions right now, inquiries, and we can't get to your question until next month. So I guess all that to say, like, why not? Why not large organizations?
Bryan Lee:I think part of that is actually more just on like startup go to market, right? the interesting thing here is the problem actually exasperates usually on both ends of the scale when you have not a lot of lawyers and when you have a lot of lawyers, because typically then you're a large organization with more volume to so actually it's ironically, you're serving either likely serve very small customers or very large customers. I have very large customer sites. It's just more down to the go to market efforts of sales cycles. I think with larger companies the self sectors are just going to be, nine months plus. And, as an early startup, we raised our. Like our large precinct just closed in June. We have to be focused on cell cycles that are shorter. Thank you. And so I think that's the byproduct of just, like startup life, right? I also think the budgets are less constrained with large companies still. So the traditional tendency is to go to outside counsel. And then you still have budget to pay for outside counsel to do that. And so there's definitely more resource constraint for smaller companies that do have to maximize on efficiency as much as possible. So I think there's still that budget shift that, that is happening. And There are more senior legal leaders that I think have not made that shift to what can I use AI for? So their default muscle is I'm going to go to the person I used to work with at the law firm and then use their services as outside counsel, right? Which is the typical legal path of how that's solved
Jed Tabernero:it's funny because what you're mentioning is the same. So I worked in an accounting organization for a long time and it's pretty similar in accounting organizations. We have all these complex use cases that we need to think through. And instead of just relying on internal resources, we'll hire PwC or we'll hire Deloitte.
Bryan Lee:Yes.
Jed Tabernero:for us because a lot of our people come from there.
Bryan Lee:So there's this interesting psychology where I think I don't know how this plays out exactly because humans still rely on like this comfort security or whatever, this notion of relationships with other humans. So I don't know if that's still this sort of like linchpin or whatnot, until we actually fully trust. And yeah, I, rely on AI in particular, I think there's still this. Human in the loop and a lot of things and that, yeah. So I'm curious what your thoughts are on that.
Jed Tabernero:I think they'll always will be. Humans will always be there and we'll probably. Always lack trust in AI for these really big projects. So I think it's really beneficial that you're always calling out that the job is more, it's a draft, right? For example the drafting piece, right? It's not creating a formal document for you, because I would never for myself, even if legal counsel didn't review this document that they had made through our internal, maybe GPT tools, right? I'm not going to trust it. I want a lawyer to look at this, right? Not just AI, right? So I think that's it's a trust break, which I'm curious to hear your thoughts on just the whole humans having trouble trusting AI, because I feel like that is, that's a huge barrier that you have to go through in convincing these very traditional, roles. And you mentioned earlier, some lawyers have PTSD from getting on board into these projects that take six to eight months, right? What are your thoughts on how do you get them comfortable? This is a really traditional industry.
Bryan Lee:So what we actually do is at different events, like more recently, just in Sacramento for the California lawyer's association, we teach like a complimentary prompting for lawyers class. We start with the basics of what is hallucination, what are LLMs? We spend a few minutes just using really elementary examples of probability like this is how, models are probabilistic, not deterministic, examples and then we just show them what you can do. In terms of prompting and chatting, and I actually break down prompting to it's like what is prompting? It's really just mastering communications with a computer. So if you're a good communicator, and I, and all the lawyers in the room were like, yeah, we're good communicators. I'm like, then you should have no problem. Prompting, I think people are like, have been like tipped off or pushed away by some of these technical terms. Cause like prompting like natural speak, natural language instructions to like, in this chat interface and then people get put off a little bit. They're like, we'll get a little, they get a little timid. And so I just break that down to you're just, it's just. You're a master communicator, which I think everyone in this room is and that starts to break things down. I'd also say that I think you have to really break down the nuance of certain types of legal work because not all of it, will be fully end to end automated because the nature of the legal work is just not binary entirely. And so the example of that is I have, two lawyers in a room and ask him about these questions. I'm actually get four answers Because it depends and then it depends on interpretation. That's just the nature of how laws constructed particularly in like commonwealth countries the u. s on the common law systems and so Interpretation is a big part. And then the next question there is Will you trust an AI interpretation over a human's interpretation or is it can be weighted enough? I think that is a Time will tell once you have more proof in the pudding right now, because we don't have enough use case. It's what it will take is it'll have to take AI interpretation that gets litigated and then enough AI interpretation gets litigated. And they're like, look, the AI interpretation was right. It got litigated. And the result is arguments. The result is what the AI also said. But that's going to take like years from now because of the litigation path, to get lawyers comfortable with, because usually that's what happens. If you look at the human way, it's like law firm partners are saying, look, here's my advice. And then it turns out to be true or whatever. It gets to my trouble or it goes to court and this is what it is. And then now you have more trust in that law firm partner because you're like, okay, he knows what he's talking about. Look at his 10 year track record. But like right now AI doesn't have that track record yet. So that I think once you start getting there, then you might get into a situation where humans still have opinions, but AI is opinion is probably better than some measure of some humans. And it may not still not be as good as some other humans where you hold that higher esteem, basically. That's my thinking of how this sort of plays out.
Shikher Bhandary:the cohort of lawyers are probably harder to change their thinking, right? But then you get a new set of lawyers who are a bit more open and then suddenly you get broad based adoption. It reminds me of the whole of like Waymo, right? we were seeing Waymo in San Francisco in 2015. And now suddenly you've got us this wave of maybe it's also age, it's being comfortable without a driver, just things that take time.
Bryan Lee:Yeah, and a lot of younger folk, like Gen Z folk, don't have driver's licenses
Shikher Bhandary:Yeah, exactly. And I was just looking at the stats. The wait list for Waymo Austin is like over a million people.
Bryan Lee:I'm on it.
Shikher Bhandary:Yeah I'm on it too. And a friend of mine got to write it last night. He shared with the group chat, everyone's even we need to do this,
Bryan Lee:so we find that even in our user base, you'll find that it's the the search ends the millennials that really can use our copilot immediately just run with it. And then they're like the testing and they then often give feedback back to their boss, who might be the general counsel or a law firm partner who's typically. Probably the older, generations of X or Boomer, and then they're the ones who are the decision makers and they actually don't go into the app to test it.
Shikher Bhandary:All you need is a Gen Z lawyer, become partner, and then you're set. Seems like that's the adoption curve.
Bryan Lee:So you need. Yeah, that's the offshoot. So it's. Likely 15 years or whatever from some 10 or 15 years for some Jetsy. But it's funny you mentioned that on adoption. Cause and I'll show this on this pod because it can be something users will find it interesting. So because of the early startup phase, of where we are, ironically, I was showing our product to finance friends and they were like, wow, I would use this immediately. And I was like, what do you mean you use this immediately? And then, cause a lot of, so a combination of finance friends corporate finance, for example, it's one use case, they often have to review the contract to just pull out. The payment terms and pull out the indemnification or limitation on liability terms. And then they model the risk. This is my, some of my friends back at some of the big tech companies, and they're not lawyers. Interpretations contract looks like I just need to pull out these terms and then put it into a spreadsheet model basically and they're like your tool does this for me and so Whereas the lawyer is still going to read through the whole contract to all this other due diligence And they're like, I just need a better control f That's like reliable and I need to pull it out. And then your tool could even tell me in plain English what does this actually mean? And so actually we're chasing this down as an experiment. And then we actually talked with my finance friends a little bit more. And cause we were actually exploring this on putting the SEC database in there with regulatory filings. And then he said that he, and some investment banking friends that we were talking to have said Oh, yeah. Like folks like that, as well as investor relations, they would use this multiple times a day to basically pull 10 K filings, 10 Q filings, and then look for the right documentation, pull it out, like I need to know what the RQ is, or I need to know who owns 5 percent of this company, et cetera And we can, what we've built is actually, we're able to do that really well. Cause we started with, Legal documents. And we could actually then look at all this, all these documents, like regulatory filings or other things like that, or earnings call transcripts as well, but actually deliver it end to end and actually solve the problem for a lot of finance professionals. And this approach was looked at, but there's still like a legal community, like M& A lawyers and capital markets lawyers that look at this information too, but actually that solves a very interesting problem. And that's something that we're actually chasing down right now.
Jed Tabernero:That's interesting because I was going to bring this up later on. I looked at one of, the products that you have called data grid
Bryan Lee:That's right.
Jed Tabernero:I work in this space. I mentioned I worked in manufacturing, right? One of the things that we do is we model what we're supposed to make and what we're supposed to buy. And guess what? I spent a shit ton of my time on with the supplier. Supplier contracts, right? These procurement contracts is looking for the damn terms. Sometimes I'm just literally looking for net 30, net 45. Like sometimes that's it.
Bryan Lee:No, you nailed it. You nailed it. So I actually think that's like a very interesting learning that we've had was that like cause most lawyers know roughly where to look for that agreement. And then somehow I can look at those payment terms. I know exactly what they say, but there's this like lawyer adjacent group, which also has to look at documentation. Or regulatory products or other things. And they don't have that same training or don't need to look at the whole contract. They just need to have a better control app, basically. And ideally one that's interpretive. That's exactly what DataGrid does. So maybe I can show you this after the call. And you can play around with it.
Jed Tabernero:think that would be interesting. I was looking at the columns and the first thing I asked myself was, why can't Excel do this? It's because I'm spending six hours making a database on Excel on these documents that I have to look one by one. Okay. What column do I look at now? Okay. That's actually the payment structure. Okay. This is actually limited liability. Okay. I'm looking at all these terms. The lawyers ain't helping me cause they're expensive. Okay. That's expensive time. I can't get their time. I gotta go by myself and pull all these contracts.
Bryan Lee:Yeah. I love that, Jed. so for us it's actually pretty, DataGrid is really intuitive. You can ask a high level question I need to pull finance terms of these contracts. Then it starts writing the column level spreadsheet questions for you. So all the prompts are already pre written. Then you can just go in and edit them based on, it'll probably understand what you're trying to pull out already Payment terms, et cetera. you can actually tune the questions to be very exact. You have a column that says pull out exactly. what the clause is you can have another column that says I just want to know the net days and it'll just say 30 days or whatnot and then i've done this with earnings calls as well where I just say how many times was ai mentioned in these earnings calls
Shikher Bhandary:Shot that stock.
Bryan Lee:No, it's very funny. i've got when I run into things like intel and nvidia
Shikher Bhandary:Nvidia.
Bryan Lee:Yeah, don't show on video and we know where Intel's going right now. But yeah, and you can even, it's really good with sentiment analysis too, and you can just say what was the sentiment, analysis for the CEO was a bullish, bearish, neutral, or, et cetera. And then I have another one that says aggregate and summarize the top like analyst questions so that I see a pattern. But again, this is where I think it's pretty powerful data in a way where it goes as precise as you want for extraction to all the way to the other end of sentiment analysis, which is. Super cool.
Jed Tabernero:it also contextualized like the data grid, for example, beyond, let's just say I'm looking for payment terms and I want to give it a bunch of procurement contracts, let's say, that's a data set. I'm training it on, but also at the same time, maybe as an accountant, I'd like to understand, what is our, Fixed asset policy, capitalization wise, like the things that are happening, which rely on maybe a per unit price negotiation or, a bulk purchase type of policy. And you're saying some of these tools are providing context and some of these tools can actually. give sources, right? And for us, we have a shit ton of documentation on just rules on everything, just common practices that we do. And maybe, I don't know, maybe this is a step further, but just to understand okay, is that, is this stuff capitalizable? The stuff that I'm buying from the supplier, so is that data contextualized? Can that be trained on some local data sets
Bryan Lee:Yeah. I think that would have to be the next phase of where we are with where data grade is. But I think that is entirely possible. What you're describing is basically like I would read this analysis. But here's like a baseline of. Knowledge documents that I want to baseline against. It actually is, it's part of this data room due diligence use case that we're looking at and yeah, so I think that's like the 2. 0 of where this would go. But right now we're, Finding a lot of demand on the finance side where we are exploring this use case as well as putting copilot directly with the SC database and transcripts earnings call transcripts to see how we can better improve that finance research use case. I think there is a stronger demand and appetite that we've seen from that space where we're just leaning into that or just think what you have to do as a startup. And yeah, we're excited to see where that goes. I'm excited to explore you, Jed, on, your latest project and how we can make that better.
Jed Tabernero:Yeah there's probably two general places that, that I would maybe just as high level suggestions. If you were looking at, other areas to go and sell to, which is one is procurement. That's one big thing. I know finance professionals we've mentioned this whole time, but procurement will benefit a ton from that, at least for us, like really large organizations. We have a really complex supply chain. All of them have different terms. So I think that's one space. And then the other space I lightly mentioned earlier is. That's a huge practice. A huge practice where a ton of folks require a lot of context. They have their internal policies that you can feed to the LLM. But there's also general policies that, come out of PwC, Deloitte. These are all public stuff. Stuff that you can train, the model on.
Bryan Lee:Yeah. I love it. I love it. Judd. Thank you.
Shikher Bhandary:We need a place to Jed was thinking of actually flying out to Austin. So we should just hang out and just talk through all the
Bryan Lee:Oh, for sure,
Jed Tabernero:be interesting to just talk through use cases.
Bryan Lee:Yeah. Let's do it.
Jed Tabernero:and there
Shikher Bhandary:people we are We could be sitting here for six hours and just keep going. So I will we want to be respectful of your time Coming to your technology stack, right? The word on the street is you have the big LLM Companies you have open AI you have Anthropic you have cohere you have all these big players Gemini and stuff What stack are you using? And do you see the need to build a custom solution? I was just reading yesterday where, building a custom solution a lot of folks are going that way, but it costs a lot of money. It's 2 million plus for a custom. built LLM because, data is an issue and engineering talent is an issue and
Bryan Lee:I think it does depend on your application. But even last night, I think that I was reading something where. initially there was a lot of flack on people building on these original models and then now they're looking at the analysis and it's look at perplexity, like it's built on, these big models and it's done really well I would say and in the legal space, you want to map things back to the product experience, for the user and what they're looking for. And I think we thought about doing custom model. The reality is we don't need a custom model to achieve the end result for the user. I think it would just, it actually adds other complications where in a lot of companies, they're actually very comfortable with open AI because their infrastructure teams have evaluated it, for example, or maybe they're already using Microsoft Copilot internally. And then so we're using, the developer enterprises over the eye doesn't train on your data. That's gets people very comfortable. Whereas we've actually heard people will have more pushback if you have a custom model where they don't understand how to test it, And they don't know. And then what's the value benefit of that custom model? It's very hard to prove what that is because opening up for legal use cases. does a pretty great job at reasoning. And then I think that extra effort we do is more on the rag layer plus classic machine learning that we can do. For each customer, which actually you see more of that last mile of results and better UI is probably where we want to invest in. So we have a full time designer on the team, which we made that investment early, and we always get compliments of our UI is really intuitive. And so I think that's where I, we're making those trade offs, but there is, at one point there will be a, like my CTO mentions that at one point there will be, it will make sense for us to build a custom model when costs will be too high for maybe using the open air model or performance issues. Cause it's just too big of a model for us and we don't really need it. And that would probably be the main reason we'd switch out. Is that rationale? But for now, I think it's okay. And even as we're looking at custom data sources, we don't have to put that at the model level. You're just putting it on this middle layer of model effectively.
Shikher Bhandary:That's great to know. And just to give the audience insight into the rag technology that Brian mentioned. So rag is retrieval, augmented generation, and it's you can think of it as a way to make AI more grounded, so the answers are more accurate with rag and perplexity is a big user of rag because it actually uses like a search database. So like how Google search works. So it's grounded with reality and that way. You reduce the risk of the hallucinations and the guessing work that has gone very viral on social media. So rag is something that's probably here to stay. And, it's pretty cool that you're, it's probably critical for you to have rag in your solution.
Bryan Lee:Yeah. Because even I think if you train a custom model here, rag will probably deliver more accurate results than your custom model, because of the nature of the LLM model, it could, It can still more hallucinate, even if you've customized it. Yeah, I agree with you that I think right here to stay until we may figure something out better. I think always fascinating to me is this idea of using, AI as judges on outputs and things like that. We have a little bit of that in our systems. But I'm interested to see more development in that space and from folks that are pushing the limits there because I think that will be continue to be really helpful Is AI as judges in the system as well.
Shikher Bhandary:That's a really interesting takeaway that commonly used LLMs like OpenAI and with RAG, with a custom RAG for your purpose. In this case, Ruli AI might in many ways be better or equivalent to a custom built LLM. This conversation has been amazing, Brian. Thanks for walking through the pain points within the legal system. We are not experts. You're the subject matter expert and walking us through where those friction points are where the inefficiencies are and where, how we, our solution can actually be used to. Make lawyers lives not as miserable as it was. I think as we wrap this call, give the founders the stage to talk about their team, talk about where the audience can find them, can learn about the new product features.
Bryan Lee:Awesome. No, thanks to you both for having me. Really enjoyed this call. It's just been super fun and thought provoking. Yeah, like where we are, like, we're not we've raised a really great pre seed I'm probably going to raise a seed sometime next year. A larger seed. We've got a pretty good handle on the legal use cases that we've built out and we're supporting on in house counsel. And we also have some large law firms that have been interested in using our copilot side. But I think as we, we touched on a little bit with Jed we're really going hard in uncovering some of these finance professional use cases. So if you're, an investment banking, private equity And or maybe corporate finance. Let us know. I would love to get in touch. Take a look at the data. Great data. Great feature. Really dot a I and as well as the copilot side as well. And let us know what you think. And if there's something we can help you out with, we'd love to just. Jam more and brainstorm with folks. If you're in Austin give a shout out to me. I'm based in Austin. My co founder is an SF. If you're in either of those places reach out, we'd love to get in touch.
Jed Tabernero:Sweet. That wraps us up.
The information and opinions expressed in this episode are for informational purposes only. And are not intended as financial investment or professional advice. Always consult with a qualified professional before making any decisions based on the concept provided. Neither the podcast, nor is creators are responsible for any actions taken as a result of listening to this episode.